Autonomous mobile robots form an important research topic in the field of robotics due to their near-term applicability in the real world as domestic service robots. These robots must be designed in an efficient way using training sequences. They need to be aware of their position in the environment and also need to create models of it for deliberative planning. These tasks have to be performed using a limited number of sensors with low accuracy, as well as with a restricted amount of computational power. In this contribution we show that the recently emerged paradigm of Reservoir Computing (RC) is very well suited to solve all of the above mentioned problems, namely learning by example, robot localization, map and path generation. Reservoir Computing is a technique which enables a system to learn any time-invariant filter of the input by training a simple linear regressor that acts on the states of a highdimensional but random dynamic system excited by the inputs. In addition, RC is a simple technique featuring ease of training, and low computational and memory demands.
Keywords: reservoir computing, generative modeling, map learning, T-maze task, road sign problem, path generation
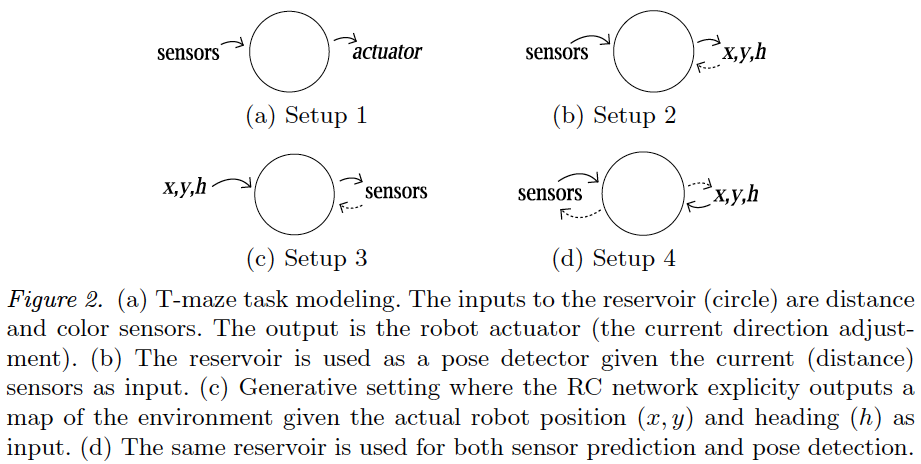
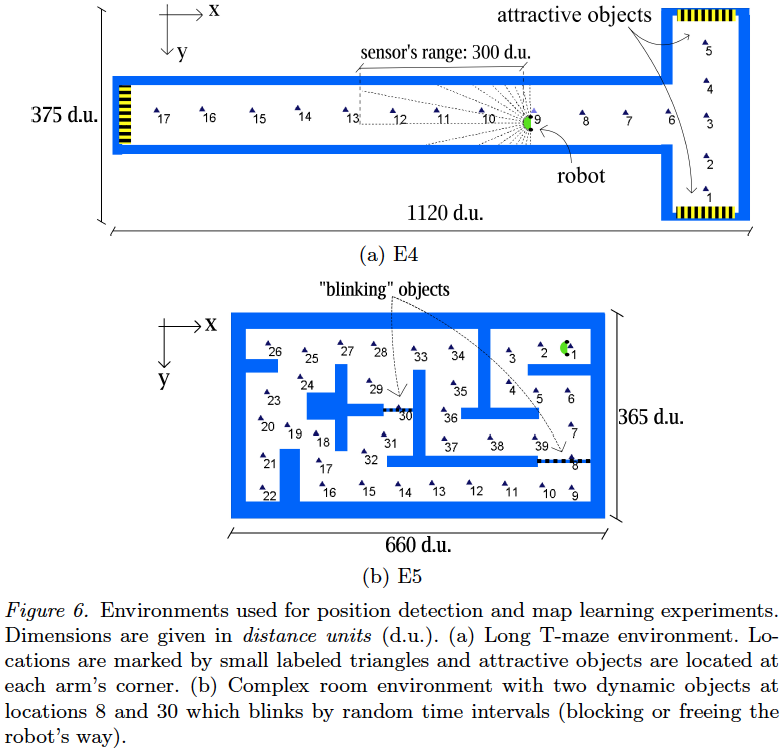
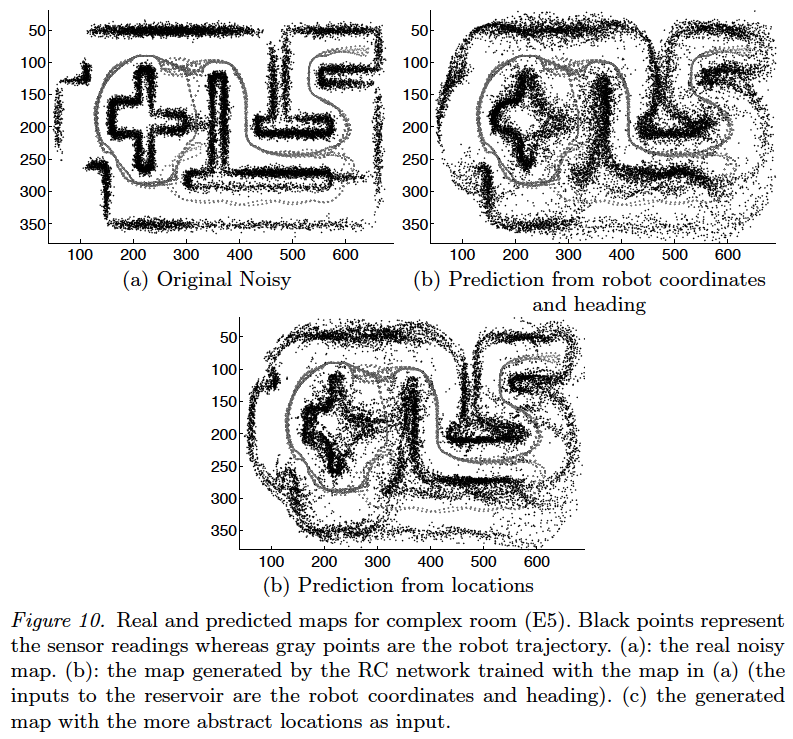
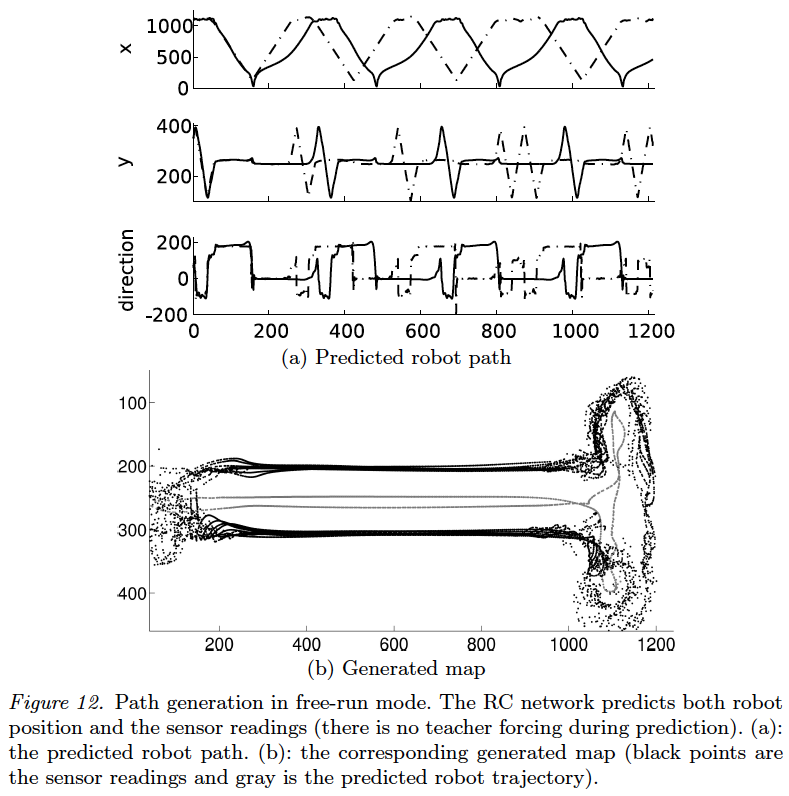
Related Publications
- Eric Antonelo, Benjamin Schrauwen and Jan Van Campenhout Generative Modeling of Autonomous Robots and their Environments using Reservoir Computing Neural Processing Letters, Vol. 26(3), pp. 233-249 (2007)
