Autonomous driving is a complex task, which has been tackled since the first self-driving car ALVINN in 1989, with a supervised learning approach, or behavioral cloning (BC). In BC, a neural network is trained with state-action pairs that constitute the training set made by an expert, i.e., a human driver. However, this type of imitation learning does not take into account the temporal dependencies that might exist between actions taken in different moments of a navigation trajectory. These type of tasks are better handled by reinforcement learning (RL) algorithms, which need to define a reward function. On the other hand, more recent approaches to imitation learning, such as Generative Adversarial Imitation Learning (GAIL), can train policies without explicitly requiring to define a reward function, allowing an agent to learn by trial and error directly on a training set of expert trajectories. % In this work, we propose two variations of GAIL for autonomous navigation of a vehicle in the realistic CARLA simulation environment for urban scenarios. Both of them use the same network architecture, which process high-dimensional image input from three frontal cameras, and other nine continuous inputs representing the velocity, the next point from the sparse trajectory and a high-level driving command. We show that both of them are capable of imitating the expert trajectory from start to end after training ends, but the GAIL loss function that is augmented with BC outperforms the former in terms of convergence time and training stability.
Images from the three frontal cameras located at the left, central, and right part of the vehicle, respectively. They were taken after the first few interactions of the agent in the CARLA simulation environment considering our defined trajectory. Each camera produces a RGB image with 144 pixels of height and 256 pixels of width. These images are fed to the networks as they are.
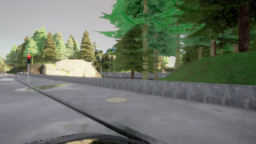
Architecture of the actor-critic network and discriminator - each of them has its own separate network, with the latter having an additional input for the action, in orange color, and a sigmoidal output $D(s,a)$ instead of the output layer of the actor-critic network which consists of the steering direction, throttle as actions for the actor (policy) and value of the current state $V(s)$ for the critic. The common, though not shared architecture (in blue) is composed of a convolutional block that process the images of the three frontal cameras, whose output features are concatenated with other nine continuous inputs for speed, next target point in the sparse GPS trajectory, and a high-level driving command. The resulting feature vector is input to a block of two fully-connected (FC) layers.
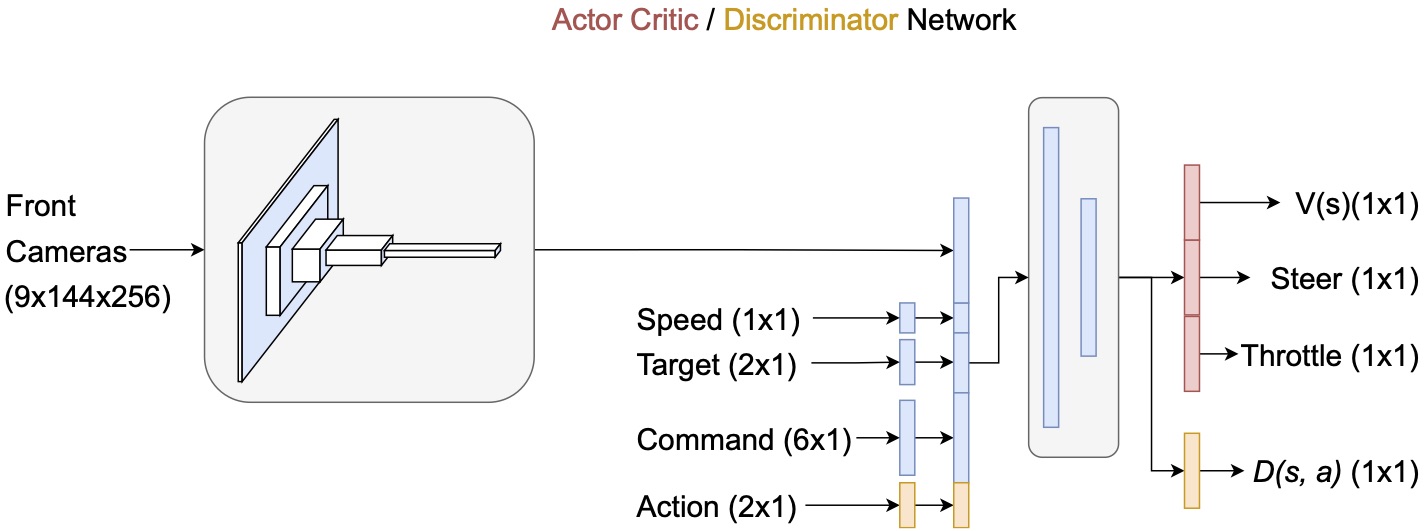
Average rewards vs environment interactions during training in the long route (setup 2). For each method (GAIL and GAIL with BC), the average performance of two runs (i.e, two agents trained from scratch) is shown with a stochastic policy (left plot) and a deterministic policy (right plot). The shaded area represents the standard deviation. The behavior cloning (BC) attains an average reward of 173.6 for ten episodes, while the maximum is at 760, achieved by both GAIL and GAIL augmented with BC.
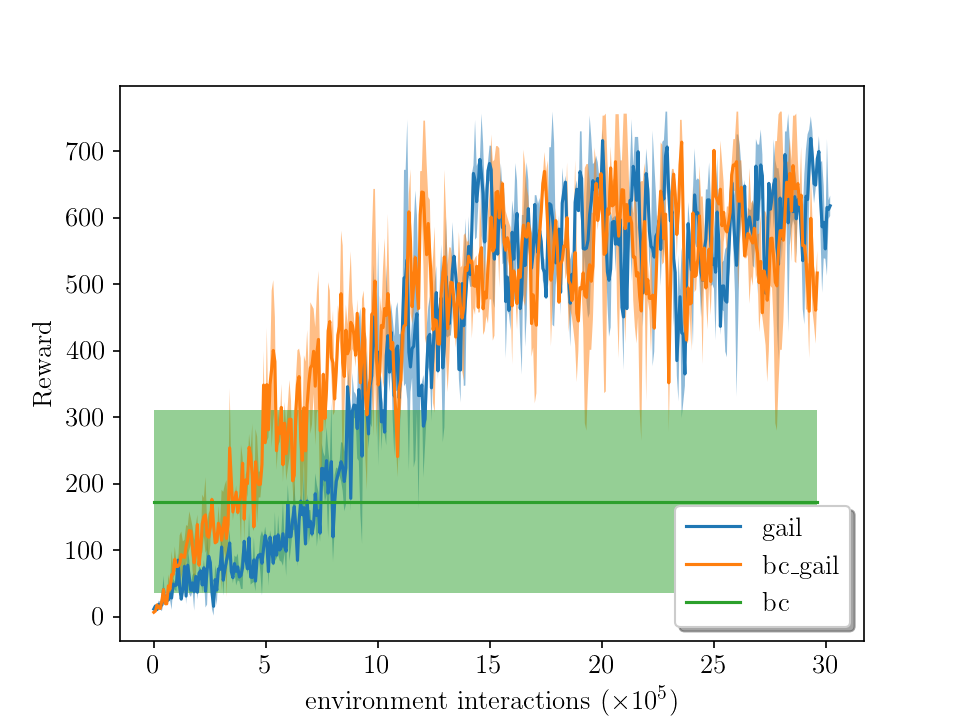
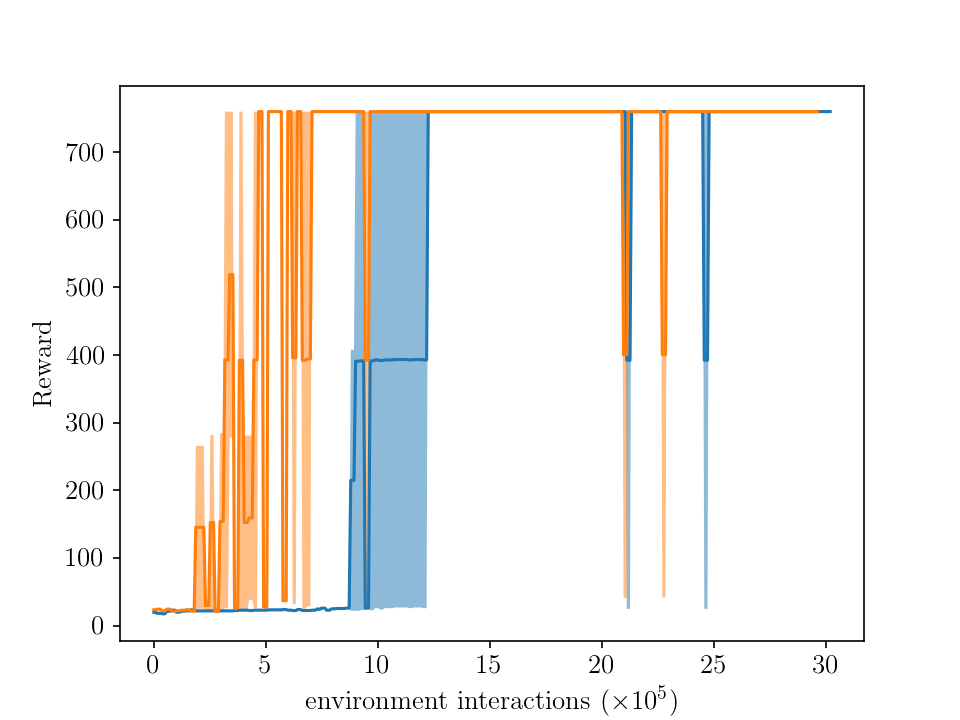